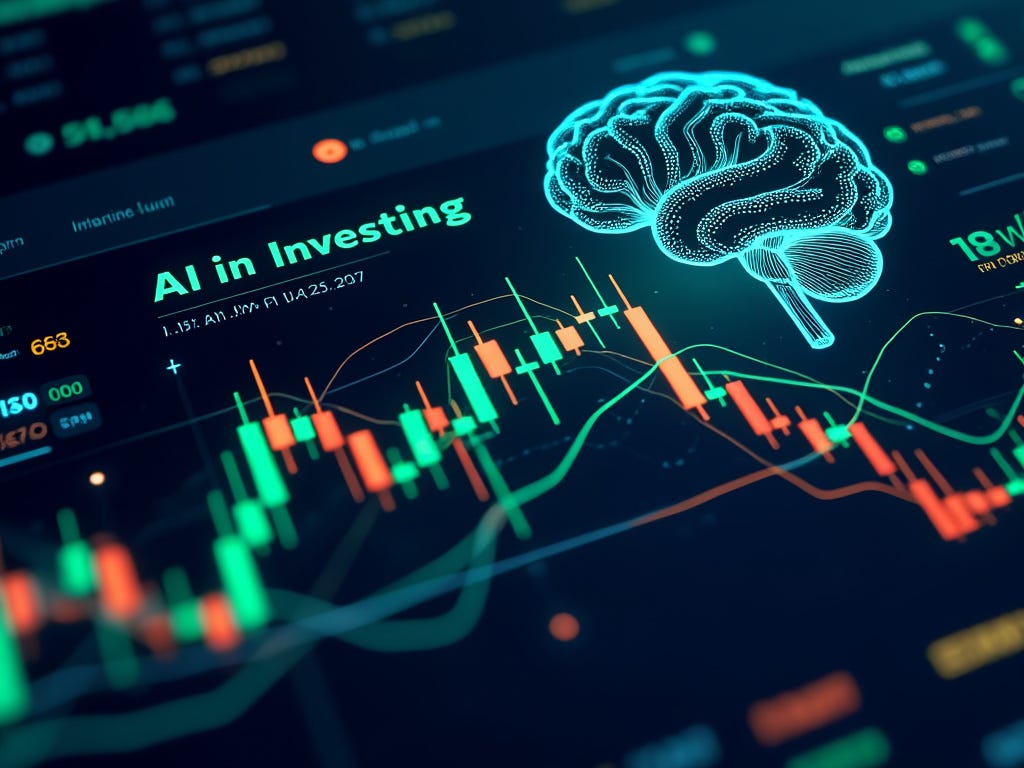
Artificial intelligence (AI) and machine learning (ML) are revolutionizing the investment management industry. From predictive analytics and algorithmic trading to risk assessment and portfolio optimization, AI-driven technologies are reshaping how investors, asset managers, and financial institutions approach decision-making.
As financial markets evolve, fund managers need smarter tools to stay ahead. CQ leverages AI to enhance investment research, risk analysis, and investor tracking, providing deeper insights from due diligence documents for more precise, data-driven decision-making.
This article explores how AI is reshaping the industry and how CQ optimizes alternative investments with intelligent automation.
Table of Contents
How AI and Machine Learning are Reshaping Investment Management
AI and Machine Learning are fundamentally altering the way investment professionals analyze data, manage risk, and execute trades. The growing reliance on AI in investment decision-making has accelerated due to the increased complexity of financial markets and the need for greater efficiency. Here’s how these technologies are making a significant impact:
- The Shift from Human-Designed to AI-Evolved Trading Models
AI is not merely automating trades, it is fundamentally reshaping quantitative finance and algorithmic trading. The era of human-designed, rule-based models is giving way to self-learning AI systems that continuously evolve, detecting hidden market inefficiencies and adapting in real time.
Self-Learning Trading Algorithms: Beyond Rule-Based Quant Strategies
Traditional quant funds rely on pre-set formulas and statistical models, which can become obsolete as market conditions shift. AI-driven trading models, however:
- Continuously adapt by processing vast, real-time financial datasets, identifying emerging trends before human analysts can.
- Use deep learning techniques to refine predictive accuracy, uncovering non-linear relationships that static models overlook.
- React autonomously to market anomalies, mitigating risks and adjusting strategies faster than traditional quant models.
From Static Factor Investing to Dynamic, AI-Optimized Smart Beta
Factor investing has long been a staple of institutional asset management, but AI is transforming it into a real-time, adaptive strategy:
- AI-driven factor weighting adjusts allocations dynamically based on changing volatility, economic indicators, and investor sentiment.
- Machine learning models analyze billions of data points, optimizing exposure to value, momentum, low volatility, and quality factors based on evolving market trends.
- Real-time alpha discovery enables AI-powered funds to exploit short-lived market inefficiencies that traditional strategies miss.
As AI continues to evolve, human intervention in trade execution and strategy development will become increasingly limited, marking a new paradigm in autonomous, AI-driven portfolio management.
- AI-Driven Alternative Asset Strategies
While AI’s impact on equities and bonds is well-documented, its rapid adoption in private markets, venture capital, and real estate is now transforming how institutional investors approach illiquid asset classes.
Real-Time Alternative Asset Valuation: Algorithmic Pricing for Illiquid Markets
Traditional valuation models struggle with private assets, as pricing relies on infrequent transactions, subjective appraisals, and historical benchmarks. AI revolutionizes this by:
- Processing alternative datasets, satellite imagery for real estate, supply chain data for private equity, and web traffic analytics for startups – to derive real-time valuations.
- Predicting fair market values by analyzing comparable transactions, macroeconomic conditions, and financial disclosures.
- Enhancing secondary market liquidity by enabling algorithmic trading and price discovery in previously opaque asset classes.
Predictive Due Diligence in Private Equity & Venture Capital
AI-driven due diligence is revolutionizing fundraising, investment selection, and LP-GP matching by:
- Assessing startup success probabilities based on historical data, founder backgrounds, and market dynamics.
- Analyzing private fund track records to predict future performance and risk-adjusted returns.
- Automating legal and financial risk analysis, ensuring compliance and reducing manual due diligence workloads.
By leveraging AI-powered alternative asset insights, institutional investors can unlock alpha in private markets, gaining greater transparency and efficiency in deal execution.
- The Real AI Edge in Risk Management: Adaptive Stress Testing & Scenario Modeling
Risk management is shifting from static historical models to AI-powered, real-time stress testing, which adjusts dynamically to black swan events, geopolitical risks, and sector-specific disruptions.
From Historical VaR Models to AI-Driven, Adaptive Tail Risk Analysis
Traditional Value-at-Risk (VaR) models rely on historical data, assuming past correlations hold in future crises. AI-enhanced models, however:
- Simulate millions of potential scenarios based on real-time economic indicators, investor sentiment, and liquidity conditions.
- Identify evolving tail risks, such as supply chain collapses, regulatory shifts, or AI-driven flash crashes.
- Dynamically adjust portfolio risk exposure, moving beyond static hedging strategies.
AI-Powered Stress Testing for Market Shocks & Systemic Risks
AI-driven scenario analysis allows institutional investors to:
- Model geopolitical and economic disruptions, incorporating real-time data from news, social media, and global markets.
- Quantify cross-asset contagion risks, forecasting how market events spread across equities, fixed income, and alternative assets.
- Build adaptive portfolio hedging strategies, optimizing derivative exposure and safe-haven allocations in response to changing market conditions.
By using AI-enhanced risk management frameworks, asset managers can proactively mitigate market shocks, ensuring their portfolios remain resilient in volatile environments.
- The Rise of AI-Driven Investor Relations & Fundraising
AI is redefining how capital is raised, transforming LP-GP relationships, fund reporting, and investor communication into an automated, data-driven process.
AI-Powered Investor Matchmaking: Smarter Capital Allocation
Raising capital is no longer about mass outreach, AI-driven investor targeting optimizes fundraising efficiency by:
- Matching fund managers with LPs based on historical investment behavior, sector mandates, and risk appetite.
- Identifying high-probability investors through AI-driven engagement tracking and capital flow analysis.
- Personalizing LP outreach, ensuring fund managers connect with strategically aligned capital providers.
Automated Fund Reporting: AI-Driven Transparency & LP Communication
AI is revolutionizing fund transparency by:
- Automating LP reporting, generating personalized, real-time investment updates based on capital commitments, fund performance, and risk metrics.
- Enhancing compliance tracking, ensuring regulatory reporting and ESG disclosures are efficiently structured and validated.
- Improving investor engagement, using NLP-powered AI to analyze LP concerns, sentiment, and feedback trends.
By leveraging AI in investor relations and fundraising, fund managers can optimize capital-raising strategies, enhance reporting efficiency, and strengthen LP relationships.
Optimize your investment workflows with AI – Discover CQ today
Challenges & Ethical Considerations of AI in Investment Management
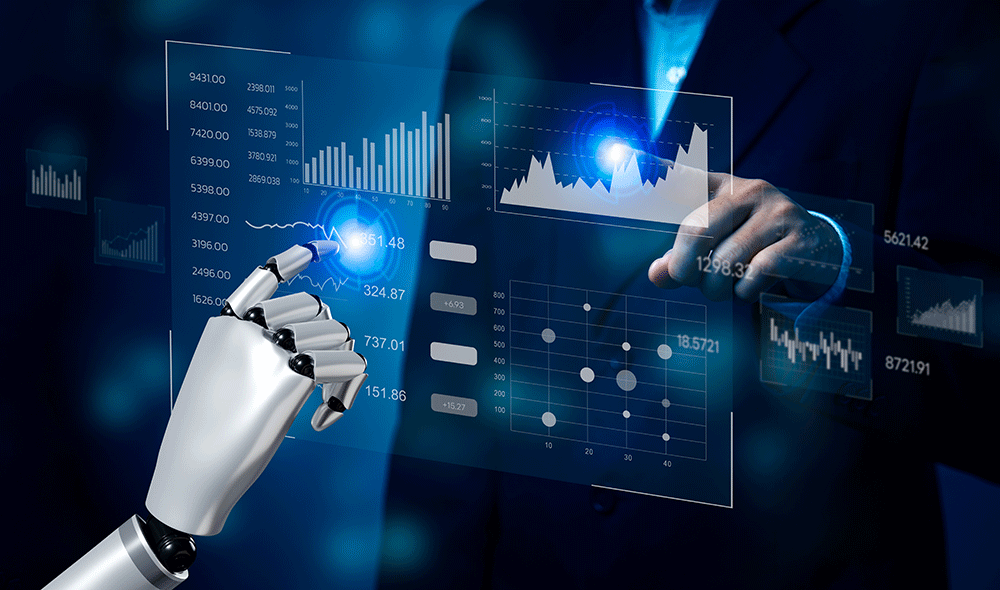
The rise of AI in investment management has revolutionized risk analysis, portfolio optimization, and trade execution, offering speed and efficiency beyond human capability. However, its adoption comes with significant challenges and ethical considerations that fund managers must carefully navigate.
1. Data Bias & Model Reliability
AI models learn from historical data, but if that data contains biases or incomplete information, it can lead to skewed predictions and suboptimal investment strategies. Some key risks include:
- Survivorship bias in financial datasets, where historical winners dominate the data, leading AI to underestimate risk.
- Overfitting to past market conditions, making models ineffective in adapting to new economic disruptions or black swan events.
- Limited transparency in AI decision-making, known as the black-box problem, where investment professionals struggle to interpret how AI generates recommendations.
To mitigate these risks, investment firms must diversify training data, continuously refine algorithms, and implement human oversight to validate AI-driven insights.
2. The Need for Human Oversight in AI-Driven Decision-Making
While AI can analyze vast datasets and detect patterns faster than any human, it lacks judgment, intuition, and qualitative reasoning. Over-reliance on AI in investment management raises concerns such as:
- Automated trading failures: High-frequency trading (HFT) algorithms have historically triggered flash crashes due to automated miscalculations.
- Market anomalies and geopolitical events: AI struggles to interpret unstructured data like political instability, central bank policies, or regulatory shifts.
- Ethical responsibility: AI-driven trading strategies may inadvertently prioritize short-term gains at the expense of long-term market stability or ethical investing principles.
The solution is a hybrid approach, where AI enhances efficiency, but human portfolio managers retain final decision-making authority, ensuring strategies align with broader financial objectives and ethical considerations.
3. Regulatory & Compliance Challenges in AI Investment Platforms
Global financial regulations are evolving to keep pace with AI-driven investment strategies, but compliance remains a key concern. Investment firms using AI must address:
- Transparency and explainability: Regulators demand clear reasoning behind AI-generated investment decisions, requiring auditability of AI models.
- Fair trading practices: AI models must comply with anti-manipulation laws, ensuring that automated trading does not distort market conditions.
- Jurisdictional differences: AI-driven platforms operating across multiple regions must comply with laws such as SEC regulations in the U.S., MiFID II in Europe, and MAS guidelines in Singapore.
To stay compliant, investment firms need robust governance frameworks, AI ethics committees, and clear documentation of AI’s role in decision-making.
4. Cybersecurity Risks in AI-Driven Investment Management
AI-driven platforms rely on large volumes of financial data, making them prime targets for cyber threats and data breaches. Critical risks include:
- AI model manipulation: Cybercriminals can exploit vulnerabilities in machine learning models, feeding them false data to manipulate market predictions.
- Investor data security: AI-driven platforms store sensitive financial records, requiring advanced encryption and cybersecurity protocols to prevent breaches.
- Algorithmic hacking: Hackers targeting high-frequency trading algorithms could cause artificial market disruptions for financial gain.
Investment firms must implement advanced threat detection, encryption technologies, and AI-specific cybersecurity protocols to safeguard both investor assets and proprietary algorithms.
Balancing AI Innovation with Ethical Investment Practices
AI is reshaping investment management, but ethical considerations remain central to its responsible use. Fund managers must balance AI-driven efficiency with human expertise, ensuring transparency, fairness, and compliance.
By proactively addressing data bias, regulatory requirements, and cybersecurity risks, the financial industry can harness AI’s potential without compromising investor trust or market integrity.
For more insight about enhancing investment management, check out Boost Your Returns With Top-Rated Investment Software In 2025
Case Study: How AI is Transforming Investment Management in Practice
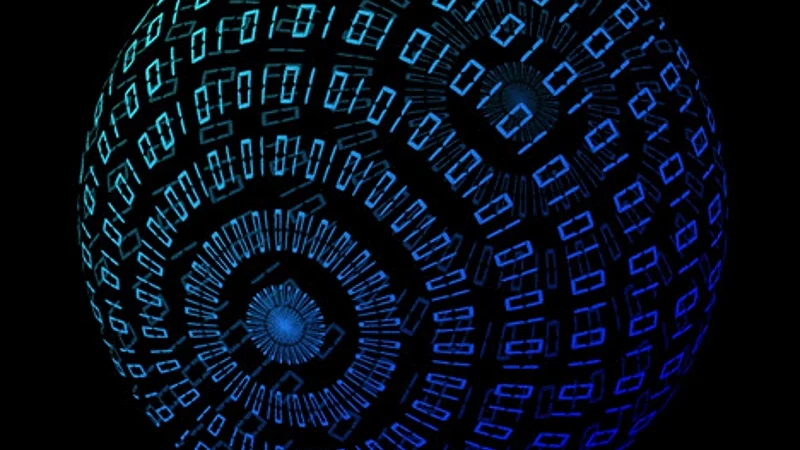
AI is reshaping investment management across hedge funds, asset managers, and private market firms, driving efficiency, automation, and deeper insights. Below are real-world examples of how leading financial institutions leverage AI, alongside CQ’s specialized approach for alternative investments.
BlackRock: AI-Powered Risk & Portfolio Optimization
BlackRock, the world’s largest asset manager with over $10 trillion in AUM, integrates AI and machine learning into its Aladdin platform, a leading risk management and portfolio analytics system.
- AI-Driven Risk Assessment: Aladdin conducts advanced stress testing and scenario analysis, evaluating portfolio responses to economic shocks, geopolitical risks, and volatility spikes. Aladdin’s capabilities allow for multi-variable risk modeling and predictive analytics across global markets.
- Real-Time Portfolio Optimization: AI helps BlackRock’s managers adjust asset allocations dynamically, optimizing for risk-adjusted returns.
- Predictive Credit Modeling: AI-driven analysis evaluates sovereign and corporate debt risks, incorporating alternative datasets such as satellite imagery and consumer trends.
Takeaway: AI-powered risk models and macroeconomic forecasts provide institutional investors with data-driven portfolio adjustments that respond to real-time market shifts.
JPMorgan Chase: AI in Trading & Fraud Detection
JPMorgan uses AI to enhance trade execution, fraud detection, and regulatory compliance across its global investment and commercial banking operations.
- AI in Trade Execution: Its proprietary trading engine, LOXM, executes large trades with minimal market impact, using self-learning algorithms that adjust execution strategies in real time.
- Fraud Detection & Compliance: JPMorgan’s AI scans millions of transactions daily, detecting anomalies and suspicious activities, reducing fraud risks across institutional portfolios.
- AI for Predictive Analytics: AI-driven models help identify potential market risks, enhancing hedging strategies and operational efficiency.
Takeaway: AI’s speed and precision improve trade execution and regulatory compliance, benefiting both institutional and retail banking clients.
Goldman Sachs: AI-Driven Private Market Investing
Goldman Sachs has invested heavily in AI for private equity, venture capital, and alternative asset strategies, recognizing the value of AI in sourcing, due diligence, and fund performance analysis.
- AI-Powered Deal Sourcing: Goldman Sachs integrates machine learning algorithms to identify high-potential private market investments before competitors.
- Automated Due Diligence: AI extracts insights from financial statements, legal contracts, and alternative data sources, streamlining deal evaluation.
- LP Engagement & Fund Structuring: AI enhances capital-raising strategies by profiling institutional investors and customizing fund structures based on data-driven insights.
Takeaway: AI accelerates deal execution in private markets, helping institutional investors identify, evaluate, and optimize capital deployment more efficiently.
How CQ Enhances AI-Driven Alternative Investment Management
While BlackRock, JPMorgan, and Goldman Sachs focus on risk management, trading, and private market execution, CQ specializes in AI-driven investment research, due diligence, and investor intelligence for alternative asset managers.
- AI-Enhanced Investor Targeting & Fundraising: CQ helps fund managers identify LPs based on historical commitments, fund mandates, and risk profiles, streamlining capital-raising efforts.
- AI-Powered Due Diligence & Compliance: CQ enables instant document analysis, extracting key fund terms, risks, and compliance insights, reducing manual effort.
- AI-Driven Investment Research: Unlike traditional AI trading systems, CQ focuses on structuring and optimizing investment intelligence, allowing alternative fund managers to make data-backed decisions faster.
For more insights about Due Diligence, check out Financial Due Diligence in Investment: Key Insights for Smarter Decision-Making
The Future of AI in Investment Management
The investment industry is undergoing a profound transformation, with AI serving as the driving force behind innovation and efficiency. Several key trends are shaping the next generation of AI-powered investment management:
1. Advancements in Natural Language Processing (NLP) for Financial Intelligence
AI-driven NLP algorithms are becoming increasingly sophisticated, enabling automated sentiment analysis of earnings calls, SEC filings, and global financial news. These advancements provide investors with real-time insights into market sentiment, corporate governance, and macroeconomic shifts, making AI an indispensable tool for decision-making.
2. AI-Powered ESG Investing for Sustainable Portfolios
The rise of environmental, social, and governance (ESG) investing has led to a surge in AI applications that assess sustainability risks. AI-powered platforms can analyze climate impact, corporate governance, and ethical investing factors, allowing investors to construct sustainable and socially responsible portfolios with higher transparency.
3. Hyper-Personalized AI-Driven Investment Strategies
Advanced AI algorithms now tailor investment strategies based on individual investor profiles, behavioral finance insights, and real-time risk adjustments. This level of customization enhances investor engagement and ensures optimized financial planning for clients of all sizes, from retail investors to institutional funds.
4. AI-Powered Private Equity Fund Management
AI is transforming private equity fund management by enhancing capital allocation efficiency, portfolio monitoring, and exit strategy optimization. AI-driven analytics provide predictive deal insights, helping fund managers make data-backed decisions with greater accuracy.
As AI technology continues to advance, investment professionals who embrace AI-driven solutions like CQ will gain a decisive edge in the competitive financial landscape.
Conclusion
AI and machine learning are transforming the investment management landscape, offering unprecedented efficiencies in data analysis, risk management, and decision-making. From algorithmic trading and predictive analytics to AI-powered due diligence and investor targeting, AI is reshaping how fund managers optimize investments.
CQ is at the forefront of this transformation, providing AI-driven tools that enhance deal flow, automate risk assessment, and streamline investor outreach.